Should Artificial Intelligence be used to Officiate Sport?
Should Artificial Intelligence be used to Officiate Sport?
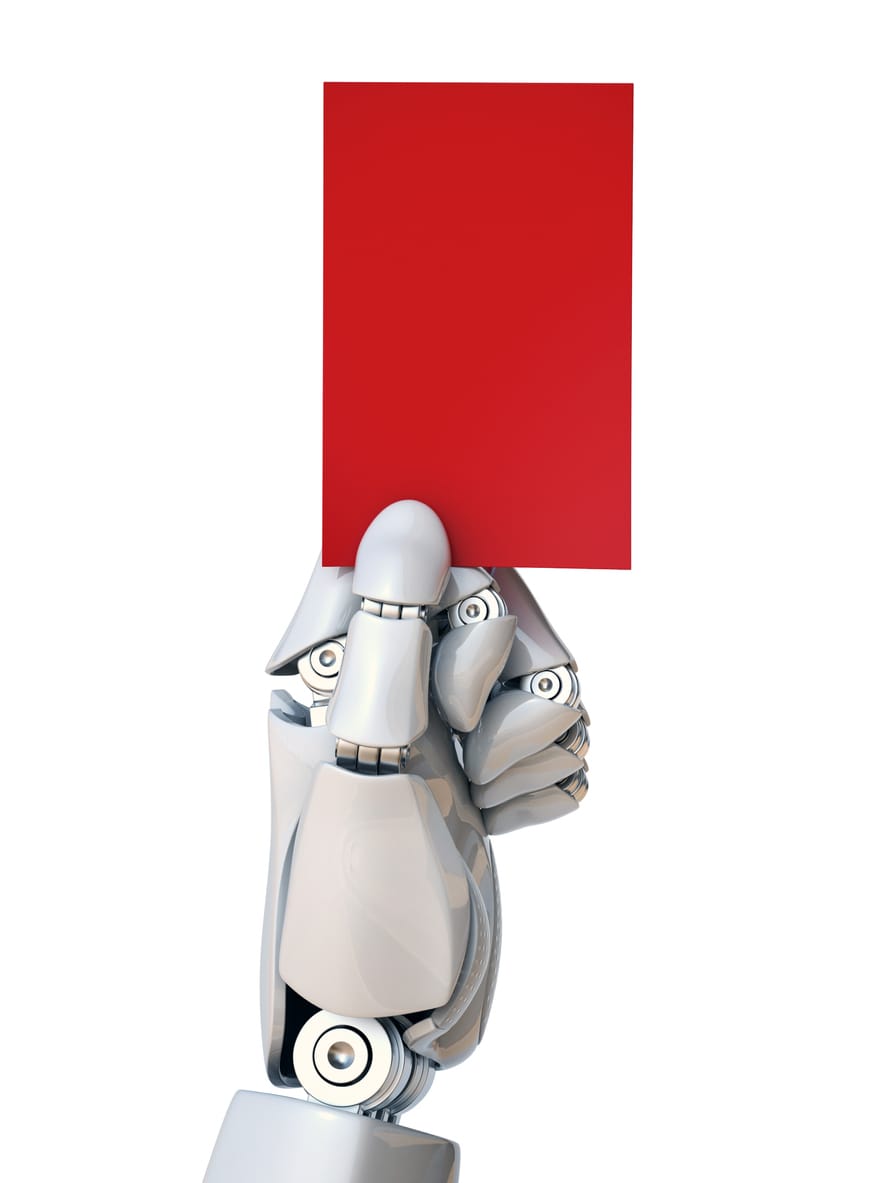
Nearly a decade ago, a study suggested a 98% likelihood that the roles of referees, umpires and other sporting officials would be computerised. In 2019, a virtual strike zone arrived in professional baseball, a roof-mounted black box is now the arbiter of balls and strikes: the TrackMan device. The International Gymnastics Federation is to introduce artificial intelligence technology to assist with scoring at the Tokyo 2020 Olympic Games (now 2021).
For some, these will be new and uncomfortable developments whilst for others, just an inevitable, natural progression of technology in sport. Notwithstanding that some sports are more readily suited to the use of technology, such developments are an inescapable progression of technological advances, and more recently, artificial intelligence (AI). Expert Andrew Ng has described AI as the ‘new electricity’, predicting the ways that we work and live will change drastically. In short, AI will have a significant impact on the world of sports.
What is artificial intelligence?
Today, AI is generally regarded as referring to using computers to do things that normally require human judgement. The online publication Quartz provide a more detailed definition:
“Artificial intelligence is software or a computer program with a mechanism to learn. It then uses that knowledge to make a decision in a new situation, as humans do. The researchers building this software try to write code that can read images, text, video, or audio, and learn something from it. Once a machine has learned, that knowledge can be put to use elsewhere.”
Whilst performing the same tasks as their human counterparts, AI-powered machines have significantly lower error ratios, and do not need to take breaks or rest. Furthermore, huge volumes of information can be processed at speed, continuously referencing and analysing old and new data.
A.I. Refereeing VS A.I. Scoring
This article specifically explores AI in officiating sports and is broken into three sections:
- AI scoring (skateboard vert example)
- AI refereeing (Formula 1 example)
- Implications of AI officiating
1. AI Scoring
Is AI needed?
Not all sporting metrics are determined by wholly objective measures such as time and distance. Max Verstappen’s qualifying lap time of 1:36.045s will not be questioned by his fellow drivers. Nor is a 100m sprinter’s time queried by other athletes in different heats. Nor an Olympic downhill ski jumper’s distance by his peers. What might be questioned is the legality of score. In other words, did the individual break any of the sport’s rules to achieve this score. But the actual score, i.e., time or distance, assigned to them is deemed/accepted to be wholly fair and accurate. These metrics do not have any bias towards any athlete.
However, not all sporting results/scores are determined by emotionless devices and performance judgment is an inherent part of sports. In fact, almost one-third of all Olympic sports use human judgment to partially or entirely assess performance. To note, the vast majority of sports rely on scores/results calculated through invented scoring systems. Putting refereeing to the side (see later), performance is ultimately assessed in objective terms: the number of points or goals scored.
Table showing how an athlete/team’s score is computed
The above table is not an attempt to classify all sports but to provide an indicative view of which sports rely on performance judgement. Combat sports such as boxing are won by knockout but if no knockout occurs, then judges determine who won the contest. Sports such as skiing have different scoring systems depending on the individual discipline. For example, downhill slalom is measured objectively with time whereas half-pipe skiing relies on performance judgement. Generally, aesthetically pleasing sports are scored by judges.
Judging the judges (human biases)
Fairness demands impartiality and thus any bias, deliberate or otherwise, is by definition a bad judgement. To date, research in sport has identified a number of different biases:
- Patriotism bias: judges favouring athletes from their own country.
- Reputation bias: judges are influenced by an athlete’s reputation.
- Rank order bias: judges giving athletes lower marks than someone competing later in the competition, irrespective of their actual performance.
- Memory-influenced bias: judges memories influence perceptual judgements.
- Conformity effect: judges are likely to adapt their own scoring to ‘fall in line’, especially when able to see the scores given by their judging peers.
There is no doubt that human bias plagues sports that require a judgment to be turned into an objective number (score). Individual sports recognise the issue, it’s just some are more proactive than others. Typical improvements have seen an increase in the numbers of judges, binning the outliers and using some form of an average, like the medium in gymnastics. However, is it reasonable to expect judges to accurately and objectively assess performance? Is it even possible? Even putting the social and time pressures aside, the highly complex, multidimensional movements athletes perform go way beyond the information processing capabilities of any human, including judges. This implies a need for simple and less complex judgements such that transparency is evident.
This year, 2020, witnessed the X Games debut a new controversial judging system in all its skiing and snowboarding contests. Previously, judges would use a detailed rubric to determine a score on a scale of 0-100 for each run (most events have about 3 runs). The new vague format is based on ‘Overall Impression’ that ranks riders not on their best runs alone, but on their performance throughout the entire contest. Of course the X Games had their reasons and on one hand they could be applauded for attempting to move away from the human bias that is rife in X-Games judging, though on the other hand it may appear desperate to ditch the old system when the new alternative is as deeply, if not more, flawed (it puts more cognitive demand on a judges’ information processing capabilities and is less transparent with its scoring criteria).
There is research that supports the accuracy of judgements made by sports judges. Even acknowledging the research which showed, at times, judges intra- and inter-related scores to be reliable, it fails to address three fundamental issues. First, reliability does not prove validity. Whilst one might expect an accurate judgement from highly-trained, experience experts who agree an outcome, it does not mean an accurate score was delivered (the experts may have been equally biased or equally incorrect with their judgement). Second, no matter how small the error of judgment, providing scores that differ for the same performance is unequitable. Third, to prove that the judges were reliable or otherwise took weeks, if not months; an exercise that cannot be completed in real-time as all the scores need to be given before analysing the data. Thus, not a suitable solution.
Furthermore, the proliferation of commercialisation and media exposure of sporting competitions, means judgements now have huge implications for the many key stakeholders involved. Incorrect decisions can bring fame and fortune to those undeserving as well as lifetime disappointments for the underserving losers too. Subsequently, these decisions have knock-on effects: for coaches who may be sacked or hired; for fans reeling at injustices such that their idol is scored incorrectly and/or unfairly; for gamblers losing and/or profiting incorrectly; and for sponsors wishing for accurate and fair judges.
How would A.I. scoring work? (Skateboard Vert example)
Real-time data is collected through sensors embedded into clothing (e.g., shoes, watches, helmet) and the skateboard for biomechanical (accelerometer, gyroscope) data recording. High-tech cameras capture a 360-degree view of the athlete at all times, with athletes finishing to the sound of a hooter (like it is today). A number (to be determined) of AI computers (i.e., judges) utilising historic and real-time data to calculate a fact-based score, which is aggregated and conveyed to the athlete, fans and competitors of the competition within seconds (or delayed, purposely, to add some suspense). A fact-based score to be determined by the level of deviation from a set of agreed parameters for a single or combination of tricks.
The illustration above is to give some indication of what a 100% AI-scoring system might look like. It is purely a matter for specific sports as to how many AI programs are deployed to assess an athlete’s performance. It would be wise to have more than one as AI programs are just algorithms written by people, and certain aspects of a skateboarder’s performance will be valued greater than other aspects, leading to some bias (perhaps). This can be limited by having the weightings agreed upon by professionals within the skateboarding community who would work alongside the computer programmer(s) (common sense).
In order to achieve the AI-scoring system above, a trick database is essential – just like computer games such as 1080 Snowboarding and Tony Hawk’s Skateboarding developed over 20 years ago! But, how would humans rank/score the difficulty of tricks without the inherent biases previously mentioned above? The answer: Comparative Judgment (CJ). In his book Human Judgment: The Eye of the Beholder, Donald Laming states, “There is no absolute judgment. All judgments are comparisons of one thing with another”.
To rank the difficulty of a skateboarding trick, as in the below illustration, a judge is shown two tricks on a screen (supported by a video and definition if considered appropriate). The judge is asked to make a comparative assessment of which trick they believe to be harder to perform (i.e., requires more skill). This process is repeated for hundreds of tricks. Now, let’s say 1000 different judges performed these comparative judgments. Not only are the tricks ranked, but the location of a trick’s difficulty on the scoring continuum is calculated. This is vitally important as differences in difficulty are unlikely to be equal thus, the distribution of scores on the continuum cannot be proportioned equally. In other words, tricks are ranked relatively not uniformly.
To highlight this, two different sets of results are displayed above. In both judgements, the Hardflip is judged to be harder than a Kickflip, but by different amounts – and this matters greatly when attributing a numerical score. In results pool A, the Hardflip was ranked three times more difficult than the Kickflip, which resulted in scores of 0.5 (Kickflip) and 1.5 (Hardflip). In results pool B, the Hardflip was judged 1.5 times more difficult than the Kickflip, resulting in scores of 1.0 (Kickflip) and 1.5 (Hardflip). To note, the scores of 0.5, 1.0 and 1.5 are fictional and would be determined by other tricks too but are to indicate the relativity of the scoring.
A potential issue is if a competitor performs a (new) trick that is not in the database (quite possible). However, most new tricks are progressions of previous ones, for example a 900 Kickflip to a 1080 Kickflip. One way to solve this is to extrapolate (a tiny bit) from previous data. The database will have assigned a score for a Kickflip, a Kickflip 180, a Kickflip 360 all the way to a 900 Kickflip. So, if an athlete performs the world’s first 1080 Kickflip, then assign a score on the same trajectory. If it’s a new combination, then just use the combination multiplier that has been assigned, plus a new trick bonus.
Now, if the new trick is truly unique and new, then one way to score it would be to award a new trick bonus (originality) which is then multiplied by an average score of a similar trick. In other words, the sensors would detect how many times the board and athlete spun and/or held the board or slid along the coping, then an average score of all those tricks would be given. The AI-scoring system would also be able to capture the style of a skater through measures such as smoothness of landing (feet and skateboard positions), flow (foot pushes used), long grabs (timed) etc.
Typically, in a 45-second run, a skateboarder performs about 10 tricks, of which some would be combinations of tricks. To place the onus on a judge to accurately compute the vast number of variables – that do influence an athlete’s score – and invariably to make the assessment from a single viewpoint is unreasonable at best. Thus, AI-scoring systems must be used for such sports. It would negate the limits of human processing capacity as well as removing the accepted bias inherent in human judgment.
2. AI Refereeing
Is it needed?
“I venture to predict, in the near future that no race of any importance will
be undertaken without the assistance of photography to determine the winner of
what might otherwise be a so-called ‘dead-heat’.”
Eadward Muybridge (a famous photographer) in May of 1882.
In horse racing, a placing judge who stood at the finish line would determine which horse had won. However, a growing dissent of voices argued for technology to provide more accurate results. The first documented use of a photo finish at a horse race was in 1881. But, due to limitations of the technology, the film would capture a horse on the inside of the track whereas a horse on the outside would still be in motion, deeming it ahead of the pack.
In the formative years of fencing, the main issue for referees was to detect whether the tip of the sword made contact with the opponent. In an attempt to detect a hit or not, three or five judges per court were required, yet, despite this, accurate judgements of fast-moving blades were not always accurate. To solve this problem electrical signalling equipment was introduced; for the epee in 1936, the foil in 1957 and the sabre in 1988.
The National Football League (NFL) were one of the pioneers in using technology in an attempt to make better (correct) refereeing decisions, introducing a replay official to monitor the game feed from within the stadium in 1985. However, the instant replay technology was removed after six seasons on being voted out by the majority of owners who stated that it delayed the game and did not produce reliable decisions. It returned in 1999.
In tennis, to help the umpire make correct decisions, up to six line officials are used to determine whether the ball lands in or out of the court. Despite this, line officials, who were ideally placed, solely staring at a line, were unable to accurately judge consistently where the ball landed. To assist the umpire, virtual imaging was introduced, displaying ball paths in an electronic view as 3d images. The system is called hawk-eye and is now used in over 80 tournaments around the world. It allows players to challenge, up to three times a set, the officials’ decisions.
Notwithstanding the limited history of technology in sport above, two common themes occurred. First, technology continues to improve and will become more prevalent in refereeing/officiating. Second, it is met with backlash. Whilst there is an inevitability of resistance with change, this perhaps could have been manged better by not introducing technology too early, i.e., been trialled and tested before, as well as educating fans on its uses and limitations. A recent example of poorly educating fans of a new technology’s purpose and limitations is the virtual assistant referee (VAR) in football/soccer.
Judging the referees
As we have seen above, the fast-moving nature of athletes and the equipment used can make it impossible for judges to accurately differentiate whether: a ball travelling at 120mph landed a mm to the left or right of a line; the tip of a weapon that is only mm wide making contact with a specific part of the body; any contact happened between two players, and if so, where did it occur (again, which side of the line); a player’s club touched the ground before taking a shot.
Now, even ignoring human information processing capacity and given eyesight better than that of an eagle, there’s a possibility – and in some sports, a strong likelihood – that the referee did not even see the incident. Visibility of the incident could have been blocked by other players or the angle of view did not allow for critical information to be seen (the referee was blindsided). Yes, some sports are harder to referee than others, with speed and the number of players being significant factors. Of course, there is sympathy, acknowledging the difficulty of the decisions needed. However, this in no reason for sports to accept this and continue as they are.
The acceptance that rules may be misinterpreted, broken and/or bent, has allowed unwritten rules to creep into individual sports. An absolute classic example in football/soccer is not giving a yellow card. Either because a foul occurred in the first few minutes of a game or if it was a first foul or it would result in a second yellow card and thus a red card. The rule book is not different in any of these scenarios. Anyone who blames the referee for sending someone off correctly, and supposedly ruining the game, has only the player to blame. The referee is there to enforce the rules and the rules are there to promote fairness and safety.
How would AI refereeing work? (Formula 1 example)
Two cars are side-by-side entering a corner and contact is made whilst both cars are cornering. The AI-referee would use sensors and GPS to determine which driver was in the wrong place and distribute a proportionate penalty – that is written in the rules – to the deserving driver by the time they have completed the lap. Quite simply really.
Throughout its history, the FIA has been guilty of a vast amount of wrong, biased and time-delayed decisions from the stewards when two cars make contact. Unsurprisingly to a Formula 1 fan, but alarming to many others, the FIA sporting regulations have no rules whilst cars are cornering except requiring drivers to remain within the track limits! (laughable). Thus, resulting in significant grey areas. So, in order for the AI-referee to work in Formula 1 a complete rulebook is needed.
This cannot be emphasised enough. Acknowledging that rulebooks will always need to be adapted, reviewed and updated along with technology, it does not excuse any sport for having blank pages in the rule book. This applies especially in Formula 1, and especially in the corners, where drivers are most at risk to themselves and their opponents. Technology in Formula 1 is already incredibly advanced with over 200 sensors on the current series of car.
Now, the common rhetoric among Formula 1 (and other motorsport) is that the driver who has the inside line to a corner has earned the right to the corner. An oft repeated but flawed principle. The inside driver at the point of entry to the corner is off the (green) racing line, meaning that they are on the dirtier side of the track as well as needing to apply a greater turning angle to the steering wheel, thus a slower speed needed through the corner. On the other hand, despite the outside car entering the corner on the racing line, it cannot just turn into the apex where the other car will be. Therefore, both drivers have earned the right to space whilst going through the corner.
For cars to go through a corner together they will have to share the normal racing line. In the above example, when entering the corner, the orange car is on the (green) racing line and then gives the (green) apex racing line to its competitor. The blue car subsequently gives the (green) exit racing line back to its competitor. Give and receive. Receive and give. Let the most skilled driver prevail and let the fans have wheel-to-wheel racing at its best: in the corners.
Give Me Space (cornering lanes)
To prevent dive-bombing – a car getting alongside another in the braking zone by out-braking themselves – the AI-referee could officiate this, including making adjustments to braking zones for individual drivers from previous laps and factor in new tyre performance.
Rulebook Being Applied by AI Referees
A significant issue with the current system is that the race stewards take time to make a decision. Way too much time! Even when, and if, a correct decision is made, it is often not until many laps later in the race by which time the car(s) that have been referred may have been involved in another incident – an incident, perhaps preventable, that should not have happened, and perhaps worse, an incident causing injury. More worryingly, many incidents are nowadays decided after the race. It’s like not sending off a player in football/soccer for a bad tackle, who by virtue of not sending off continues to impact the game, but worse still, could injure another player or score or save the winning goal or an opposition player is sent off for injuring them.
The AI-referee would eliminate this drastic time-delayed decision, but more importantly remove any bias the stewards/FIA have towards individual drivers and/or teams as well as the courage to make decisions on race-defining actions. There are many examples of this (see Has the FIA lost control of track limits? (Part 2: Races)). A significant example in recent years was when Nico Rosberg and Lewis Hamilton collided in their opening lap in the 2016 Spanish Grand Prix. As the incident happened on a straight whilst both cars were accelerating, the above rule is in place (i.e., the FIA actually had a rule for this). But yet again, the stewards waited till after the race to make a decision, in doing so giving the drivers the opportunity to influence them.
Having heard extensively from both drivers and from the team, the Stewards determined that Car 6 had the right to make the manoeuvre that he did and that Car 44’s attempt to overtake was reasonable, and that the convergence of events led neither driver to be wholly or predominantly at fault, and therefore take no further action. The race stewards view hours after the race.
So, despite Rosberg breaking the rule (not leaving a car’s width on a straight when the other car had a significant portion of his car alongside the rear wheel of the car) with evidence proving this with no doubt, the race stewards failed to enforce their own rules. This is actually made worse when the car on the receiving end is forced out of the race (from 2nd). AI-refereeing would have simply enforced that Rosberg was at fault and given a penalty accordingly. No ifs or buts. None whatsoever.
For the stewards to hold the view that they are going to be told reliable information from drivers after the race when they are only protecting themselves, is nothing short of delusional. Not that it matters anyway, as the conclusive evidence is illustrated above, but even Rosberg later admitted that he moved ‘too late’. When was this exactly? When he was retired! Did he say that when meeting the stewards after the Spanish race?
For those who are still arguing that it was a racing incident and that it happened so fast is not sufficient. Professional sports move quickly. Just because a defender tries to honestly tackle Messi, but is late, because Messi was too quick and skilful, does not mean that the defender did not commit a foul. In Formula 1, most fans want to see wheel-to-wheel racing, yet if these scenarios are not refereed correctly, then it is going to deter a racer from overtaking/wheel-to-wheel racing. Again, notwithstanding that it was in fact a rule at the time, drivers should be rewarded (i.e., space) for being able to challenge a car in front as overtaking is extremely hard in similar cars.
As a result of this, a decision is then needed whether or not to assist the drivers on the location of other cars. The excuse ‘I didn’t see him’ is not fit for purpose in two ways. First, failing to know where your opponent is a lack of skill in itself. Second, if you are unaware of the exact location of your competitor, you should assume he is there rather than not (just like you would on a road). So, should drivers be informed by a light on the screen highlighting which part of the track they must give their competitor space? And what about lane markings on apexes and exits (like pit entry and exit lines)? Cars could have a line marked on their car (half way) so cameras and drivers can use to judge whether they are entitled to space.
Ghost Car Simulations (Ferrari engine scandal)
Anyone playing a racing game online will be familiar with a ghost car concept. AI-refereeing would provide a ghost car for all cars during the race to detect, and prevent, any unusual behaviours. In the 2019 season, teams were suspicious that Ferrari’s straight-line speed had increased significantly throughout the season. This prompted the FIA to provide a rule clarification at the US Grand Prix in October reiterating that the mandatory fuel-flow meter cannot be tampered with in any way, thus maintaining the maximum permitted rate.
This rule clarification ended Ferrari’s run of six consecutive pole positions, the team failing to achieve poles in the remaining two races. The Ferrari team principle insisted that the apparent differences in the car’s straight-line performance and the rule clarification were unrelated and due to the team adding more downforce, and therefore, drag, in its attempt to improve cornering performance. After the season was complete, the FIA admitted it was unsure whether Ferrari’s engine was always legal in 2019 and that they had reached a confidential settlement with Ferrari.
The complete lack of transparency between the FIA and the other teams is in no doubt evidence of bias in officiating Formula 1. All teams, except the Ferrari powered teams of Alfa Romeo and Haas, questioned the integrity of last year’s finishing order. Formula 1 is run on numbers, and therefore determining whether a car/team is breaking the rules, is simply – humans can do that! In itself, a ghost car may not be able to prove Ferrari’s engine legality but when viewed in conjunction with the huge amounts of data captured from a Formula 1 car, a truer picture would have emerged.
AI-refereeing should account for 95% of all decisions in Formula 1 by 2024. On provision of a full set of rules with area/cornering lane mapping in place, then applying penalties for drivers who break the sporting rules becomes a binary decision. No need to wait to hear for drivers’ explanations or 10 laps to make a decision. Most of all, bias towards individual drivers and teams is removed and credibility restored to race and championship defining decisions. Finally, ghost cars would help detect and thus deter illegal misdemeanours by teams.
3. Implications of AI in Scoring and Refereeing
A potential clash of ideologies will come to fruition. Those, including this article, who believe that AI has the capability to eliminate the flawed decision-making process and deliver wins/successes on sporting merit and those who believe inherent controversial decisions to be a fundamental part of sport. To highlight this view, decision-makers treasure the sense of unfairness in football. The International Football Association Board (IFAB) explains its aim is ‘not to achieve 100% accuracy for all decisions’ but to seek to swiftly remedy clearly identifiable mistakes in match-changing situations – namely penalties, goals and sendings-off.
How do you define match-changing situations? Surely, all actions influence a match, thus are match-changing. What about the butterfly effect? Notwithstanding such questions, a big concern for some is that less controversial moments in sport will mean less debate amongst fans, pundits and the media. Even a correct decision can still evoke emotions and debates, thus feelings of injustice may not be removed completely, and this may be a good thing. Whilst acknowledging a correct decision, the closeness of a call which sees your idol miss out in a career-defining match by a mm will still hurt and provoke interaction.
These two ideologies may be traced back to the purpose of sport: to entertain or to find the worthiest winner. Whilst there are arguments for a balance between these two views, a proponent of total AI-officiating would point out two counter arguments. First, AI-officiating would remove some element of luck involved, thus generating more matches/games between the best teams/players. Second, sport would still be entertaining, in that, most, sport is unpredictable, and luck will always be intrinsically intertwined. For example, a shot in football might hit a defender and deflect in.
Finally, the typical push back against technology is the loss of jobs and change. Looking at the former, there is no denying that referees/judges/officials will lose their current role to AI-systems, in particular amongst the elite level of sport. That is no reason in itself not to pursue better decision making in sport. However, the cost of AI technology, may mean it will not replace officials completely (certainly not in the near future). These professional officials could officiate at either lower level competitions or grassroots. For example, in English football, a tiny 1.1% of the Premier League’s annual revenue could finance 1500 referees, at a generous £35000 each year.
Summary: AI Scoring VS AI Refereeing
Sports have different needs: invasion games would significantly benefit from AI-refereeing whereas judgemental sports would significantly benefit from AI-scoring systems.
The Stat Squabbler says:
- The information processing capacity of the human brain is limited. It certainly cannot be expected to accurately score highly complex, multidimensional movements, especially from a limited viewpoint, i.e., once and/or blindsided.
- Humans and bias are intrinsically linked, consequently AI-systems must be used to deliver objective decisions, in particular, outcome-defining decisions, no matter who is on the winning or losing side of the decision.
- AI systems must be built with experts in both computer science and the sport itself. They must be trialled and tested before integration, fans educated on the changes and what to expect and its limitations.
- Despite arguing what comes first, entertainment or sport, absolute fairness and the correct results/decisions must be the goal with new technologies exploited for further (or replaced) entertainment.
Do you agree with the Stat Squabbler: Should AI be used to officiate sport? Which sports need it most? Comment below.